Key Takeaways
- Understanding the differences between data warehouses, data lakes, and relational databases is crucial for effective data management and analysis.
- Snowflake’s popularity can be attributed to its cloud-based architecture, scalability, and ease of use for managing and analyzing large volumes of data.
- The stock price of Snowflake Inc reflects the market’s confidence in the company’s potential for growth and innovation in the data warehousing space.
- Snowflake is used for various purposes, including data warehousing, data lakes, and data engineering.
- Exploring job opportunities related to Snowflake data warehousing can provide insights into the skills and expertise in demand within the industry.
- Examining examples of successful data warehouse software, such as Snowflake, showcases the impact of modern technology on data management and analytics.
- Key Takeaways
- Data Warehouse vs Data Lake
- Why is Snowflake So Popular
- Snowflake Inc Stock Price
- What is Snowflake Used For
- Snowflake Data Warehouse Jobs
- Snowflake Data Warehouse Stock
- Data Warehouse Software Examples
- Summary
- Frequently Asked Questions
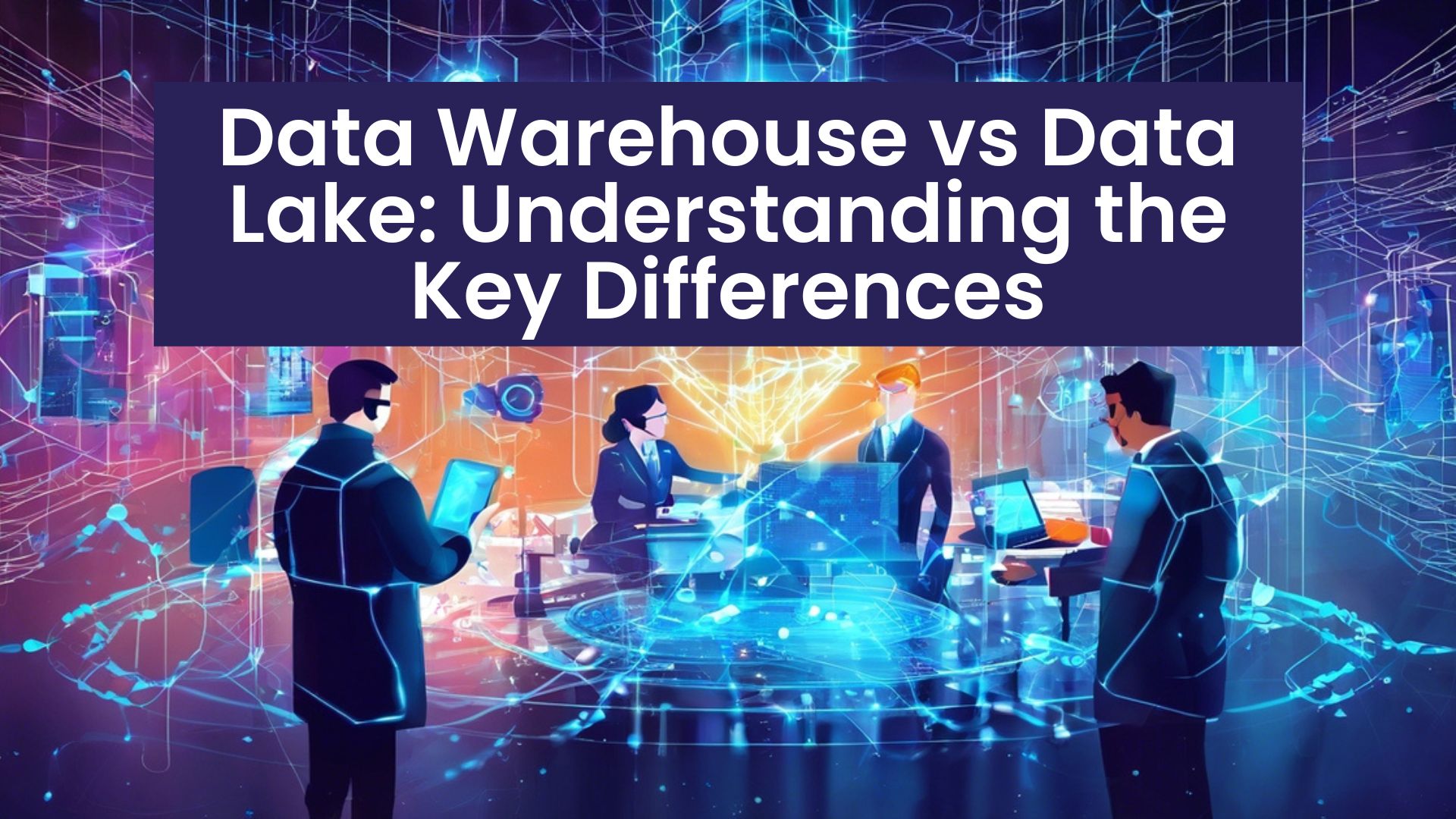
Curious about the clash between data warehouse and data lake? Confused about which one suits your business needs better? Whether you’re a tech enthusiast or a business owner trying to navigate the complex world of data storage, this breakdown will provide valuable insights that can shape your decision-making process.
Are you ready to unravel the mysteries behind these powerful tools and gain clarity on how they can impact your organization’s data strategy and lake? Let’s dive in and demystify the battle between data warehouse and data lake once and for all.
Data Warehouse vs Data Lake
Structured and Unstructured Data Storage
Data warehouse is tailored for storing structured and processed data, while data lake is designed to house raw and unstructured data sets. Imagine a data warehouse as an organized, well-kept library where each book has a specific place on the shelf. On the other hand, picture a data lake as a vast ocean where different objects are scattered around without any particular order.
Data warehouses are like carefully labeled file cabinets in an office, with everything neatly sorted into folders and drawers. In contrast, data lakes resemble a messy room filled with various items piled up haphazardly. This distinction highlights how data lakes accumulates raw data in its original form, while the other methodically arranges it for easy access.
Both data lakes and data lakehouse systems cater to different types of information storage needs within an organization; however, they serve distinct purposes based on the nature of the stored content.
Business Analytics vs Data Science
Typically used for business data and analytics requirements, data warehouses provide structured insights that help organizations make informed decisions about their operations. Think of this as analyzing sales reports, customer demographics, data lakes, or data lakehouse to improve marketing strategies or streamline production processes.
On the other hand, data lakes are often utilized in more exploratory fields such as data science, enabling researchers to delve deep into raw information to uncover hidden patterns or develop innovative solutions. It’s akin to exploring untouched terrains in search of new discoveries rather than following established paths.
Consider how these two systems function like separate departments within an organization: one focuses on optimizing existing procedures through meticulous analysis, while the other ventures into uncharted territories seeking groundbreaking revelations.
Why is Snowflake So Popular
Snowflake’s Cloud-Based Platform
Snowflake has gained immense popularity due to its cloud-based platform and data lakes. It provides top-notch security and consistency for big data analytics. This means that users can trust the platform to handle large volumes of data securely and reliably.
Snowflake is known for supporting a wide variety of data sources and applications, enabling businesses to gain valuable insights from different types of data. This versatility allows companies to use various tools and systems, including data lakes, with Snowflake, making it a highly adaptable choice for their analytical needs.
Flexibility and Scalability
One key reason behind Snowflake’s popularity is its flexibility and scalability. These attributes make it a preferred choice for data science and analytics tools, as they require platforms that can adapt to evolving requirements.
The platform’s ability to scale seamlessly allows businesses to manage growing amounts of data without compromising performance or security. As companies continue to expand their operations, having a scalable solution like Snowflake becomes crucial in ensuring smooth operations without any setbacks.
Snowflake Inc Stock Price
Enhanced Security
Snowflake Inc’s cloud-based data storage solution has surged in popularity due to its enhanced security features for enterprise data and analytics needs. The platform offers a secure environment for businesses to store and analyze their valuable data without compromising on safety.
Businesses can confidently manage sensitive information, such as customer details or financial records, within the Snowflake platform. This enhanced security feature ensures that critical company data remains protected from unauthorized access or potential breaches.
Moreover, Snowflake’s architecture allows for seamless integration of various data sources, enabling businesses to derive comprehensive insights while maintaining the highest standards of security.
Scalability and Cost-Effectiveness
One of the key factors contributing to Snowflake Inc’s stock price surge is its scalability and cost-effectiveness. The platform provides businesses with a scalable solution, including data lakes, that can adapt to their evolving analytics needs without incurring exorbitant costs.
For instance, companies experiencing rapid growth can seamlessly expand their analytics capabilities within Snowflake without facing significant infrastructure constraints or excessive expenses. This scalability empowers organizations to efficiently handle increasing volumes of data generated by diverse analytics tools while optimizing operational costs.
Snowflake’s cost-effective approach makes it an appealing choice for businesses seeking a reliable yet budget-friendly solution for managing their diverse analytics tools effectively. By minimizing unnecessary expenditure associated with traditional on-premises solutions or complex infrastructure setups, Snowflake enables companies to allocate resources more strategically towards enhancing their analytical capabilities.
What is Snowflake Used For
Data Storage and Processing
Snowflake is a cloud-based data warehouse that provides a secure storage solution for various types of data. It is used for storing and processing vast amounts of data, making it an essential tool for organizations dealing with large datasets. With its cloud-based architecture, Snowflake offers scalability and flexibility in managing diverse data sources.
Snowflake is commonly utilized across different industries for data analytics, machine learning, and business applications. For instance, companies can use Snowflake to analyze customer behavior patterns, perform predictive analysis, or generate business intelligence reports based on the stored data.
Advanced Data Security Features
One of the key advantages of using Snowflake as a storage solution is its advanced data security features. These features ensure that sensitive information and data lake remain protected from unauthorized access or breaches. Organizations can rely on Snowflake to adhere to stringent security protocols while handling their valuable data assets.
In addition to providing robust security measures, Snowflake also supports various analytics tools and platforms for efficient data analysis. This enables users to seamlessly integrate their preferred tools into the platform and leverage them for deriving valuable insights from the stored data lake.
Snowflake Data Warehouse Jobs
Managing Large-Scale Data Sets
Snowflake data warehouse jobs involve managing and analyzing big data sets within large organizations. These jobs require professionals to use various analytics tools to extract valuable insights from the vast amount of data stored in the Snowflake data warehouse. For instance, a professional in this role might be tasked with identifying trends or patterns within sales figures, customer behavior, or market trends by using specialized software for analysis and data lake.
Professionals working in Snowflake data warehouse jobs often need a strong foundation in data science and machine learning. This is because these skills are crucial for driving effective business data analysis, which ultimately aids companies in making informed decisions based on their stored information. For example, they may need to develop algorithms that can predict future consumer behaviors based on historical purchasing patterns.
Meeting Analytics Needs
The primary purpose of Snowflake data warehouse jobs is to meet the analytics needs of organizations by providing a robust solution for storing and organizing their enterprise-level data. By leveraging the capabilities of Snowflake’s architecture, professionals can ensure that businesses have access to real-time analytics and reporting functionalities while maintaining high levels of security and scalability.
These roles play a critical part in ensuring that businesses can effectively utilize their accumulated information through advanced techniques such as predictive modeling, sentiment analysis, or even creating interactive dashboards for improved data visualization. This allows decision-makers within an organization to gain meaningful insights into various aspects of their operations without being overwhelmed by complex databases.
Snowflake Data Warehouse Stock
Efficient Data Analysis
Snowflake Data Warehouse is a leading cloud-based data storage solution that caters to various enterprise data needs. It provides a platform for big data analytics, enabling efficient analysis and visualization of business data. With Snowflake, organizations can manage large volumes of structured and semi-structured data, supporting their analytics needs effectively.
Data warehouses like Snowflake offer consistent and high-quality data for analysis, ensuring that businesses make informed decisions based on accurate information. These platforms also support advanced analytics tools, allowing users to derive valuable insights from their data sets. For example, companies can use Snowflake’s capabilities to perform complex queries on massive datasets without compromising performance or scalability.
Big Data Management
In the realm of big data management, Snowflake stands out as an effective solution for storing and processing vast amounts of information. Unlike traditional databases, which may struggle with the scale and complexity of big data, Snowflake’s architecture is designed to handle these challenges seamlessly. This makes it an ideal choice for organizations dealing with substantial amounts of diverse datasets.
A key advantage of using a robust data warehouse like Snowflake is its ability to maintain data consistency, ensuring that all users access the same version of truth when analyzing business-critical information. By leveraging features such as scalable computing power and storage resources, businesses can efficiently process large volumes of both structured and unstructured data within a single environment.
Data Warehouse Software Examples
Purpose of Data Warehouse Software
Data warehouses such as Snowflake, Amazon Redshift, and Google BigQuery are designed for structured data storage solution and analysis. They cater to the needs of business intelligence and analytics tools, providing a reliable platform for storing and processing enterprise data sets.
These software solutions are commonly used with relational databases to handle big data and support data visualization for analytics needs.
For example, Snowflake is known for its ability to efficiently manage large volumes of business data while offering seamless integration with various analytics tools. Similarly, Amazon Redshift is recognized for its scalability in handling complex analytical queries on large datasets.
Benefits of Data Warehouses
- Efficiently manages large volumes of business data
- Seamless integration with various analytics tools
- Scalability in handling complex analytical queries on large datasets
In addition to these benefits, Google BigQuery stands out for its serverless architecture that allows users to analyze massive datasets quickly using SQL queries. These examples highlight how different data warehouse software provides unique advantages tailored to specific business requirements.
Importance in Analytics Infrastructure
The significance of data warehouses lies in their role as a foundational component within an organization’s analytics infrastructure. By centralizing structured enterprise data, they empower businesses with the ability to perform advanced analytics such as predictive modeling, machine learning algorithms, and statistical analysis.
Furthermore, utilizing a robust data warehouse enables businesses to gain valuable insights from historical trends through comprehensive reporting and ad-hoc querying capabilities. This not only supports informed decision-making but also enhances overall operational efficiency by streamlining access to critical business information and data lake.
Summary
In conclusion, understanding the difference between a data warehouse and a data lake is crucial for making informed decisions about data management. Snowflake’s popularity stems from its ability to efficiently handle diverse data workloads, making it a valuable tool for businesses. Whether it’s for managing stock prices, handling various data warehouse jobs, or exploring software examples, Snowflake offers a robust solution. As you delve into the world of data management, remember that choosing the right platform can significantly impact your operations and insights.
So, take the time to weigh the benefits of each option carefully. Your data strategy can be a game-changer for your business, and embracing the right tools will set you on the path to success.
Frequently Asked Questions
What is the difference between a data warehouse and a data lake?
A data warehouse is like a structured, organized library, storing processed and summarized data for easy access. In contrast, a data lake is more like a raw, unstructured storage pond for vast amounts of diverse data in its original format.
Why has Snowflake gained popularity?
Snowflake’s popularity stems from its cloud-based architecture that offers scalability, performance, and ease of use. It allows users to store and analyze large volumes of data with speed and efficiency.
How does Snowflake impact stock prices?
As an investor darling in the tech industry due to its rapid growth and disruptive technology, positive news about Snowflake’s performance or market expansion can drive up its stock price.
What are some common uses for Snowflake?
Snowflake is commonly used for analytics, business intelligence reporting, machine learning applications, and secure sharing of real-time insights across organizations.
Are there specific job roles related to working with Snowflake Data Warehouse?
Yes! Job roles such as Data Engineers, Database Administrators (DBAs), Data Analysts/Scientists often work with Snowflake to manage databases, optimize queries or build analytical solutions. Familiarity with SQL is usually required.
POSTED IN: Computer Security